Deep learning models track river extents at sub-meter resolutions
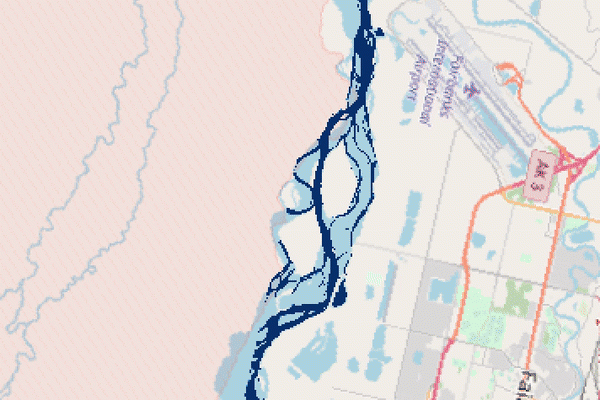
Earth’s surface water, such as river flow paths and inundated floodplains, evolves spatially over time. Where and how much surface water is present is critical for evaluating droughts, floods, and climate feedback. Remote sensing techniques have been able to track river extents at > 10-meter resolutions. New research led by Prof. Joachim Moortgat now brings such capability to a new horizon—tracking river changes at sub-meter resolutions.
Recently published in Remote Sensing of Environment, the study shows fully convolutional neural networks could identify surface water from multispectral and panchromatic imagery, which outperforms many other state-of-the-art techniques. “Tracking river changes at such a high spatial resolution was possible because the deep learning models not only capture how reflective the water bodies are but also learn the morphological features of rivers,” said Prof. Moortgat.
The project was contributed by multiple co-authors in the School of Earth Sciences: Prof. Michael Durand, Prof. Ian Howat, and alumnus Dr. Chunli Dai. “Our application on Arctic rivers will contribute to a better quantification of discharge from snowmelt”, said Prof. Moortgat, “We see potentials in scaling up the approach to a larger region using meter-scale satellite imagery, which will help narrow down uncertainties in hydrological budgets”.
Temporal changes of Arctic rivers tracked using deep learning (animation credit to Prof. Moortgat).
This project demonstrates the potential of leveraging artificial intelligence (AI) to address questions in Earth Sciences. Prof. Moortgat said, “We are working on building applicable pipelines of similar AI approaches for our community to support innovative research in Earth Sciences using AI.”
–Yanlan Liu